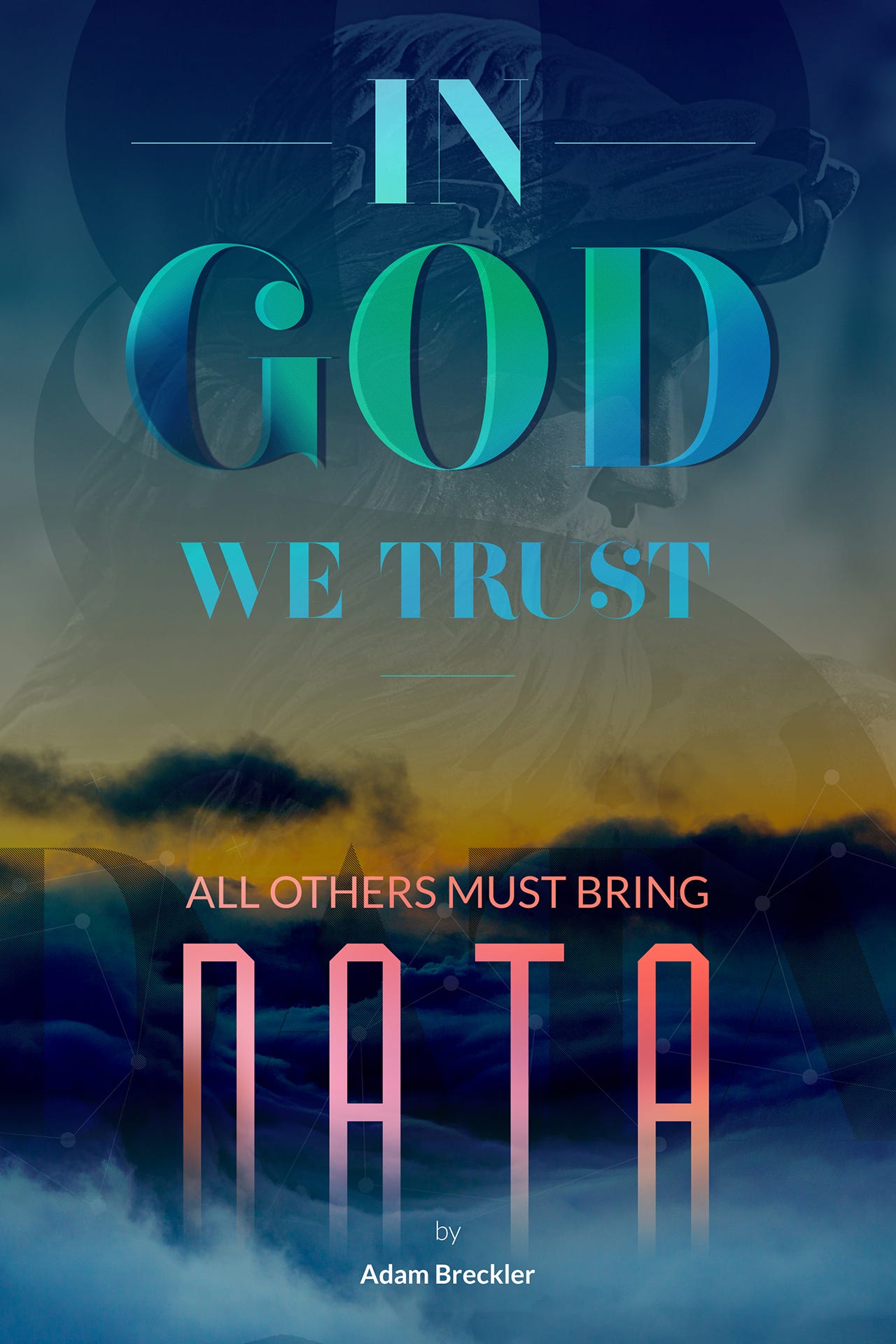
“In God we Trust, all others bring data”
Designing a Data-Informed Decision Process with Edwards Deming: Grandfather of the Lean Startup

Adam Breckler Nov 18, 2013 · 5 min read
The late William Edwards Deming was an American statistician, professor, author, lecturer, and consultant.
He is perhaps best known for the “Plan-Do-Check-Act” cycle popularly named after him, and is also credited for the quote:
“In God we trust; all others must bring data.” — [30]
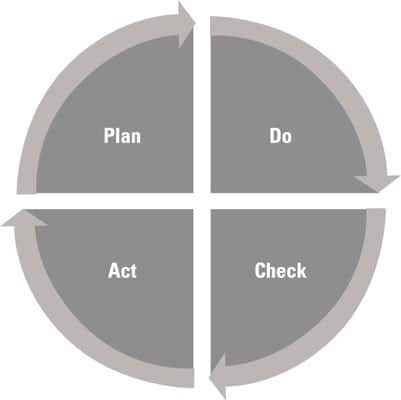
Deming’s PDAC cycle comes from the scientific model of forming hypotheses and then testing them, and it follows these steps:
- Plan.
Establish your objectives and how you plan to achieve them. In the scientific method, the equivalent step is creating your hypothesis and prediction. - Do.
Implement the plan — you make it happen. In the scientific method, this step is the test of your hypothesis. - Check.
Measure to determine what happened. The scientific method calls this step the analysis. In the internet software world, this is often put into practice with A/B testing. - Act.
Think about root causes that may explain the differences between actual and planned results. To close the cycle of improvement, act on a new plan to implement and test these root causes. This stage reflects the scientific method’s commitment to evaluation and improvement.
Every startup these days claims to be data-driven.
Most would agree that ‘data-driven decision making’ is far superior to other forms of making decisions. However, the key to using data to inform decision-making doesn’t lie in the ‘data’ part of the equation in isolation.
The hard part is quickly and effectively turning data you collect into useful information that gets translated into Understanding, Knowledge and Insights…that eventually generates better decisions…on an on-going and repeatable basis.
Data should act as the foundation for your decision-making process, not as a substitute for your own judgement. Different tools and techniques are required to deal with each step along the way.
Know the difference between data-informed and versus data-driven.
“There is no substitute for knowledge.” — E. Demings
This statement is in hypothetical contrast to the old adage, “There is no substitute for hard work” — Thomas Alva Edison (1847–1931).
Often times, a very small amount of knowledge could save many hours of hard work. Sometimes, it can save months. On rare occasions, it can even save years.
Knowledge in the context of a Startup company can be loosely defined as “That which we believe to be true about our customer value proposition that we can verify with data to be true“. This type of knowledge and understanding is paramount in the early days of a Startup’s life.
This knowledge can be derived from both Qualitative & Quantitative measures:
Early Customer development for a product or feature within a product is like qualitative research, it is good for exploring hypotheses (and associated issues) but cannot validate them (by its very nature it is small sample size).
To validate a hypothesis one needs to do quantitative research, e.g. surveys, measurements (A/B tests that test a big change, not just button color), and have an appropriate sample size.
“The most important things cannot be measured.”— E. Demings
The reality is that many of the issues that are most important, long term, cannot be measured in advance.
The corollary to this statement in the context of Startups would be:
“The most important product features can not be arrived at from continual a/b testing and optimization exercises.”
However, these might be the very factors that an organization is measuring, but are just not understood as most important at the time.
Testing different button colors on your homepage is not going to change the trajectory of your product or company in any meaningful way, and tends to lead you towards alocal maximum.
Statistics can be a powerful tool for turning data into insight but when you are trying to measure something for which there isn’t a scientifically valid approach to dealing with, you are wasting your time.
So, how then should you try and test for something which you haven’t built?
Say for example that you are trying to measure a customer’s desire or willingness to pay for a new product or feature. You can simply implement an experiment with a fake button, testing customer’s behavior.
Other techniques you can use to get you out of a ‘local maximum’ rut are:
- Repositioning the product for a stronger value proposition
- Going after a different kind of audience to target their needs
- Recalibrating the “core mechanic” of the product to make a new feature a natural part of using the product
“Experience by itself teaches nothing.” —E. Demings [29]
In other words: Data, without context, is not knowledge.
Knowledge is best taught by a master who explains the overall system through which experience is judged; experience, without understanding the underlying system, is just raw data (noise) that can be misinterpreted against a flawed theory or view of reality.
This problem can get compounded when dealing with “big data”.
“Big data can tell us what’s wrong, not what’s right.“ — N. Taleb
This is why you should be very skeptical of any “big data” analyst or “data scientist” claiming to be able to explain a system in a particular domain without the requisite domain expertise or intimate knowledge of the underlying system under consideration.
“You can expect what you inspect.” — E. Demings
Popularly known as “what you measure, you manage”, this quote from Demings illustrates the importance of measuring and testing to predict typical results.
If a phase consists of inputs > process > outputs, all three are inspected to some extent. Problems with inputs are a major source of trouble, but the process using those inputs can also have problems.
By inspecting the inputs and the process more, the outputs can be better predicted, and inspected less. Rather than use mass inspection of every output product, the output can be statistically sampled in a cause-effect relationship through the process itself.
So, how do you know you are on the right track?
When in doubt, you can always fall back on using very basic comparables for testing that are publicly available aided by simple back of the envelope math in order to be able to tell if you are on the right track towards achieving a desired outcome.
When end up almost inevitably off the mark it will be obvious (often by orders of magnitude) where you to optimize for the levers of growth in your business that produce the maximum possible leverage.
Thanks to Damian Madray.
WRITTEN BY
Adam Breckler
Internet Professional, Currently: Founder, Prism Labs (Prism.io) Previously: Co-founder & VP Product @ Visual.ly
Fonte: https://medium.com/@adambreckler/in-god-we-trust-all-others-bring-data-96784d01e9be Acesso em 12/08/2015 às 18:09